Table tennis has served as a benchmark for robotics research since the 1980s. Recently, Google DeepMind researchers have created an AI-driven robotic arm that can compete in the game at the level comparable to amateur human players. This robotic agent can adapt to an opponent’s moves in real-time, allowing for enjoyable table tennis games with human players.
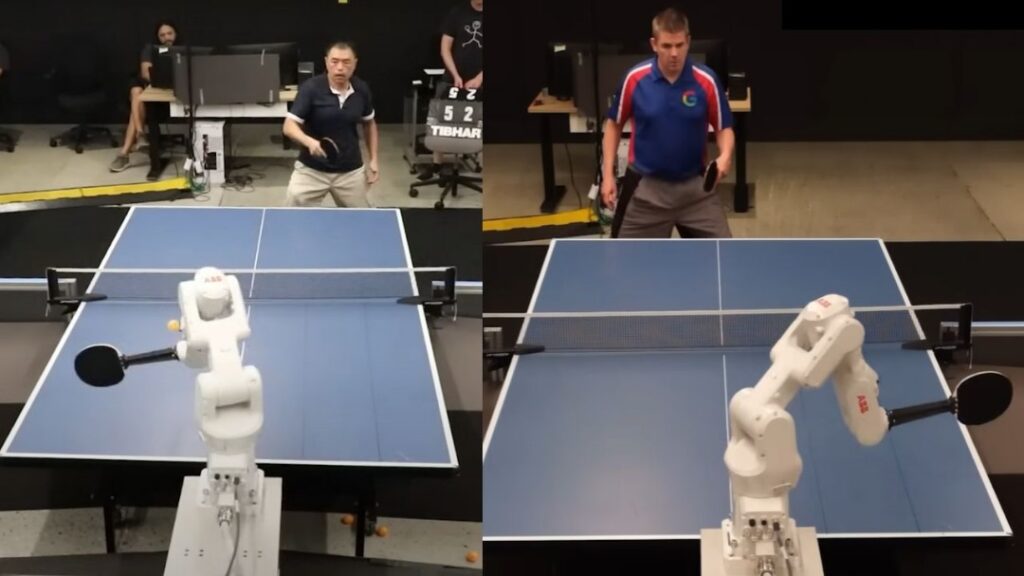
The development process began by gathering a small dataset of human versus human gameplay to set initial parameters. The AI was trained on vast amounts of data to refine its playing style, adjust its tactics throughout matches, and collect performance data. This data was then used to refine its skills in simulation, creating a continuous feedback loop. This iterative training-deployment cycle not only enhanced the robot’s performance but also tailored the gameplay complexity to mirror real-world conditions, considering the robots’ need for speed, precision, and adaptability in the sport.
The robot lost all its matches against more advanced players, who were able to exploit weaknesses in the robot’s policies. However, the robot won all its matches against beginners and 55% of games against intermediate players.